In the fast-paced world of product development, where agility and innovation are paramount, trust serves as the bedrock upon which success is built. As a Chief Product Officer, I’ve witnessed firsthand the transformative power of trust within agile teams. It fosters collaboration, empowers individuals, and accelerates the delivery of valuable products.
One of the most influential books I’ve encountered on this topic is Stephen Covey’s “The Speed of Trust.” Covey eloquently argues that trust is not merely a soft skill but a tangible asset that can significantly impact organisational performance. By building trust, teams can reduce friction, increase efficiency, and achieve remarkable results.
Trust is particularly crucial; it enables teams to operate with a high degree of autonomy and transparency. When team members trust one another, they are more likely to share information openly, collaborate effectively, and make decisions that align with the team’s goals.
Transparency fosters a culture of openness, where team members feel comfortable sharing ideas, concerns, and challenges. This leads to better decision-making, problem-solving, and knowledge sharing. When team members take ownership of their work, they are empowered to drive innovation, efficiency, and job satisfaction.
Additionally, trust is essential for maintaining a high velocity of delivery. When team members trust each other’s abilities and intentions, they can collaborate more effectively, reduce bottlenecks, and deliver products faster.
But what happens when you don’t have trust? Artificial intelligence (AI) systems, while powerful, are often complex and opaque, making it difficult to understand their decision-making processes. This lack of understanding can erode trust and create significant challenges.
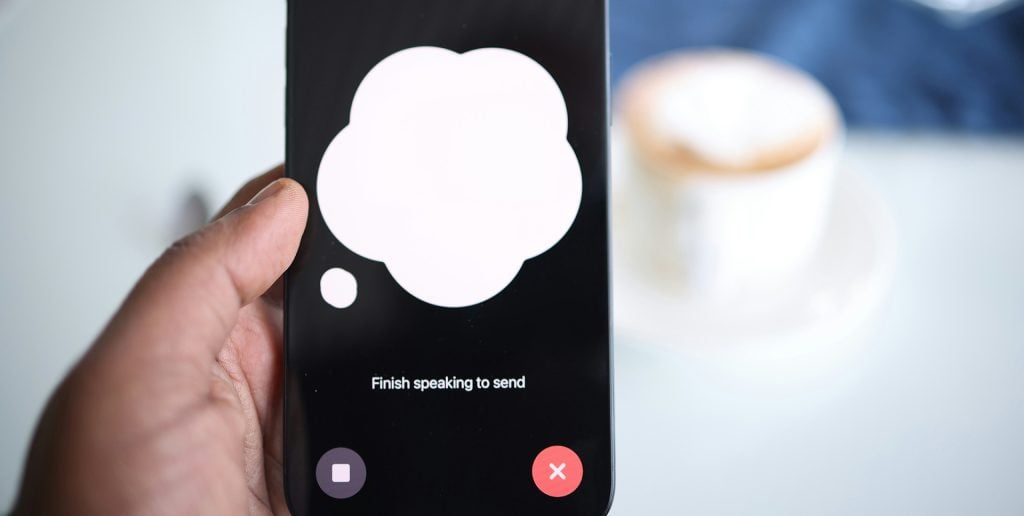
The AI trust gap
The AI trust gap is the gap that exists between the potential benefits of AI and the level of trust that people have in these systems. This gap is closed when a person is willing to entrust a machine to do a job that otherwise would have been entrusted to qualified human users.
In the context of financial services, bridging this trust gap is particularly critical. Investment managers and other regulated financial institutions often face significant challenges in gaining approval for AI projects from regulatory and approval committees.
Back at Funds Congress earlier this year, Martin Moeller, Director of Artificial Intelligence and Generative AI at Microsoft, declared that “the financial services industry is leading the way in Gen AI adoption, but how do we go about getting an application live in production in today’s regulated environment with so many unknowns and such little trust?
Build the framework
Gaining approval for AI projects in a regulated financial services environment requires a strategic and methodical approach. Start with why and create a business case that clearly defines the project’s purpose and the value it will create for your customers and other stakeholders. This approach ensures a clear understanding of the desired outcomes and the potential impact on various groups.
Establish or adapt existing governance and approval processes to address the organisation’s trust concerns. This involves creating clear governance structures, including well-defined roles, responsibilities, and decision-making processes. Implementing oversight mechanisms will enable ongoing monitoring and evaluation of AI systems.
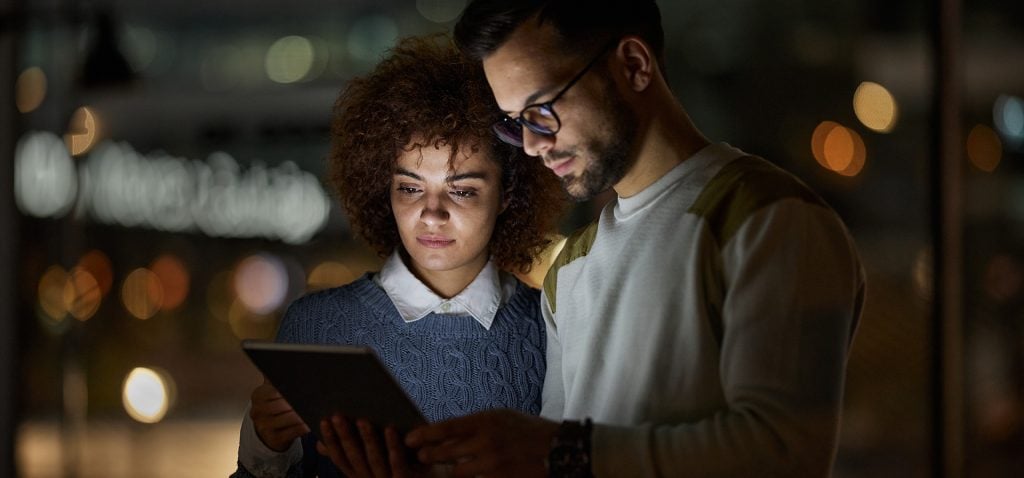
Understand the regulations and engage early
Thoroughly research and understand the specific regulations that apply to your AI project. While the global regulatory landscape for AI is still evolving, several key regions have taken significant steps to address the challenges and opportunities presented by AI technology.
In the UK, the Financial Conduct Authority (FCA) has issued guidance on the use of AI in financial services. The FCA’s approach is principles-based, allowing for flexibility while ensuring that AI is used responsibly.
In Europe, the General Data Protection Regulation (GDPR) imposes strict data privacy and protection requirements that apply to AI systems used in financial services. Additionally, the EU’s AI Act, once finalised, is expected to include specific provisions for AI in financial services, such as risk assessment, transparency, and accountability.
In the US, the Securities and Exchange Commission (SEC) has issued guidance on the use of AI in investment management. Other US regulators, such as the Commodity Futures Trading Commission (CFTC), may also have relevant oversight over AI use in financial services.
To successfully navigate the regulatory landscape, it is essential to establish a dialogue and engage with regulators early in the AI project. By seeking guidance and addressing concerns proactively, asset managers can build strong relationships with regulators and ensure compliance with evolving regulatory requirements. Furthermore, collaborating with regulators to develop best practices for AI adoption can contribute to a more favourable regulatory environment for the industry.
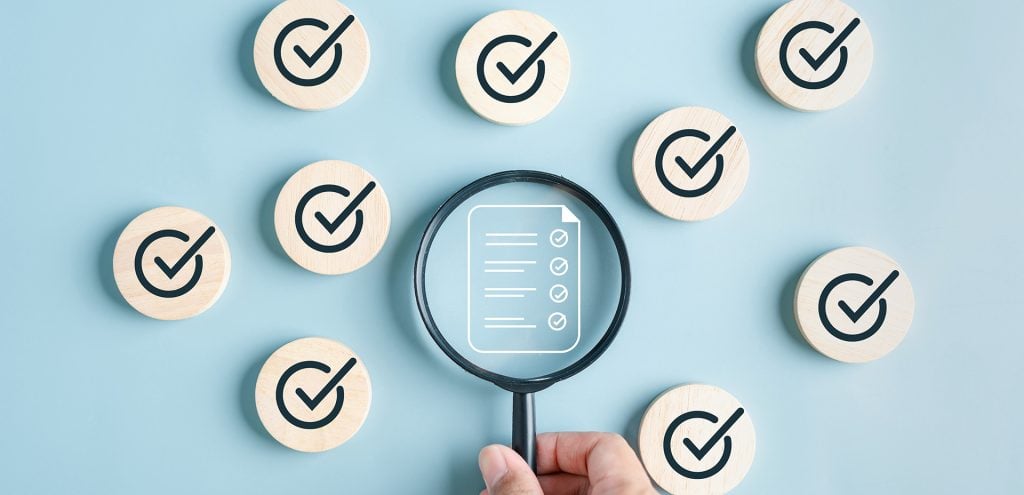
All AI is not created equal
The level of risk inherent in an AI system is determined by the type of application, the use case, and the source of data. For example, an AI system used for critical decision-making, such as credit scoring or fraud detection, will require a higher level of risk mitigation than an AI system used for customer service chatbots. Artificial Intelligence (AI) encompasses a broad spectrum of technologies, each with its own level of complexity and risk. It is critical for the decisionmaker to understand the technology they are working with.
- Machine Learning (ML) is a subset of AI that involves training algorithms on large datasets to identify patterns and make predictions. Machine learning models can be vulnerable to biases in the training data, leading to unfair or discriminatory outcomes. Additionally, these models may struggle to explain their decision-making processes, making it difficult to understand and assess their reliability.
- Deep Learning (DL), is a more complex form of machine learning that utilises artificial neural networks with multiple layers. Deep learning models can learn complex patterns from data, but their complexity also makes them a challenge to understand and assess. This can increase the risk of unintended consequences or biases.
- Generative AI, made popular by Chat GPT in early 2023 can generate new content, such as text, images, or code. This technology can be used to create highly realistic synthetic data that may be difficult to distinguish from real data. This could lead to various issues, including fraud, misinformation, and deepfakes.
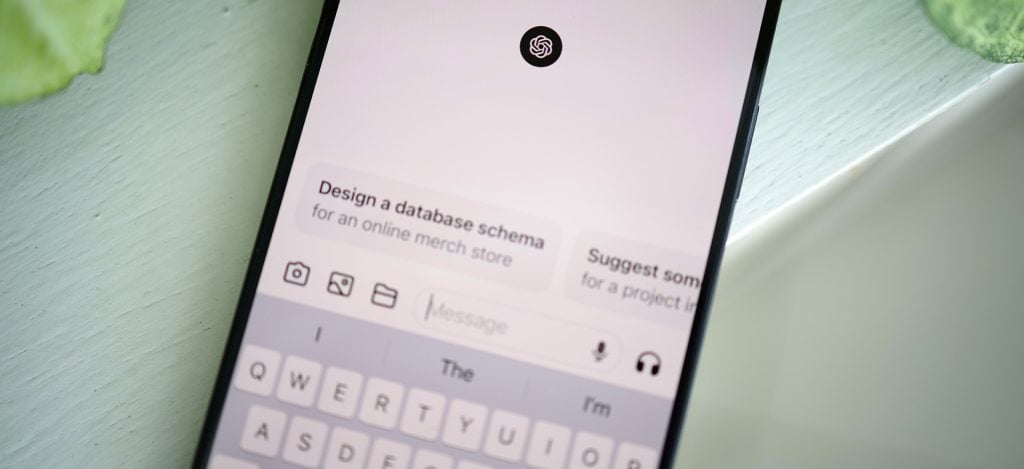
Understand and mitigate common AI risks
While AI offers immense potential, it’s essential to understand the risks associated with its deployment and implement effective mitigation strategies to minimise its potential downsides.
- Bias in decision making is one of the primary concerns. AI systems can inadvertently perpetuate or amplify existing biases present in the data they are trained on.
Mitigation: Use diverse and representative datasets, conduct regular audits, and employ techniques like explainable AI to identify and address biases. - Disinformation is a significant threat posed by AI. AI-generated deepfakes and misinformation can spread rapidly online, undermining public trust and democratic processes.
Mitigation: Implement robust content moderation strategies, support fact-checking initiatives, promote digital literacy education, and require AI-generated content to be clearly labelled. - Safety and security are paramount when deploying AI systems. These systems can be vulnerable to malicious attacks, such as adversarial attacks and data poisoning.
Mitigation: Implement strong security measures, train AI models to be resilient against adversarial attacks, and conduct regular security audits and vulnerability assessments. - Explainability is another critical concern. The black box nature of many AI models makes it difficult to understand how they arrive at their decisions.
Mitigation: Employ explainable AI techniques, analyse feature importance, and use visualisation tools to make AI models more transparent. - Ethical concerns are also prevalent in AI. AI systems can raise ethical questions related to discrimination and privacy violations.
Mitigation: Develop and adhere to ethical guidelines, ensure diversity and inclusion in AI development teams, and incorporate privacy considerations into AI design and development. - Instability is another potential challenge. AI models can be sensitive to small changes in input data, leading to unpredictable or unintended outcomes.
Mitigation: Conduct rigorous testing, train AI models to be resilient against adversarial attacks, and maintain human oversight. - Hallucinations are a known issue with AI language models, which can generate false or misleading information.
Mitigation: Train AI models on high-quality data, validate AI outputs, and have human experts review AI-generated content. - Unknown unknowns are another challenge. AI systems may have unforeseen limitations or risks that are not yet fully understood.
Mitigation: Stay updated on AI research and advancements, conduct scenario planning, and maintain human oversight.
By proactively addressing these risks, asset managers can ensure that AI is a valuable tool for enhancing their investment processes and ensuring its safe and responsible deployment.
Navigate the AI landscape with trust and expertise
We recognise the importance of building trust in a regulated environment both within your organisation and with your stakeholders. In summary we recommend the following five key steps to addressing the key risks and challenges associated with AI:
- Establish a robust governance framework: Ensure your organisation has the necessary controls and oversight mechanisms in place.
- Navigate the regulatory landscape: Understand and comply with relevant regulations, both domestically and internationally.
- Mitigate AI risks: Identify and address potential risks such as bias, explainability, and security.
- Build trust with stakeholders: Demonstrate transparency, accountability, and ethical considerations in your AI initiatives.
- Deliver successful AI solutions: Leverage AI to drive innovation, improve efficiency, and enhance customer experience.
The rapid pace of technological change can feel overwhelming, but embracing innovation doesn’t have to come at the expense of reliability or security. By taking a balanced and thoughtful approach, it’s possible to harness AI’s potential while maintaining trust and compliance. If this topic resonates with you, especially in the context of data centralisation, data visualisation and client experience, we invite you to explore more insights on our blog or connect with us directly—we’re always keen to discuss ideas and share perspectives.